Deep Learning
Deep learning, a machine-learning method aimed at achieving artificial intelligence (AI), has recently received much attention. It is hoped that by having a multilayered neural network learn a vast amount of data, versatile intelligence like that of humans can be created.
- Just as babies learn words, AI itself learns and makes judgments
- Extracting relationships from large amounts of labeled data using multilayer neural networks
- More applications for consumers as vast amounts of data become readily available
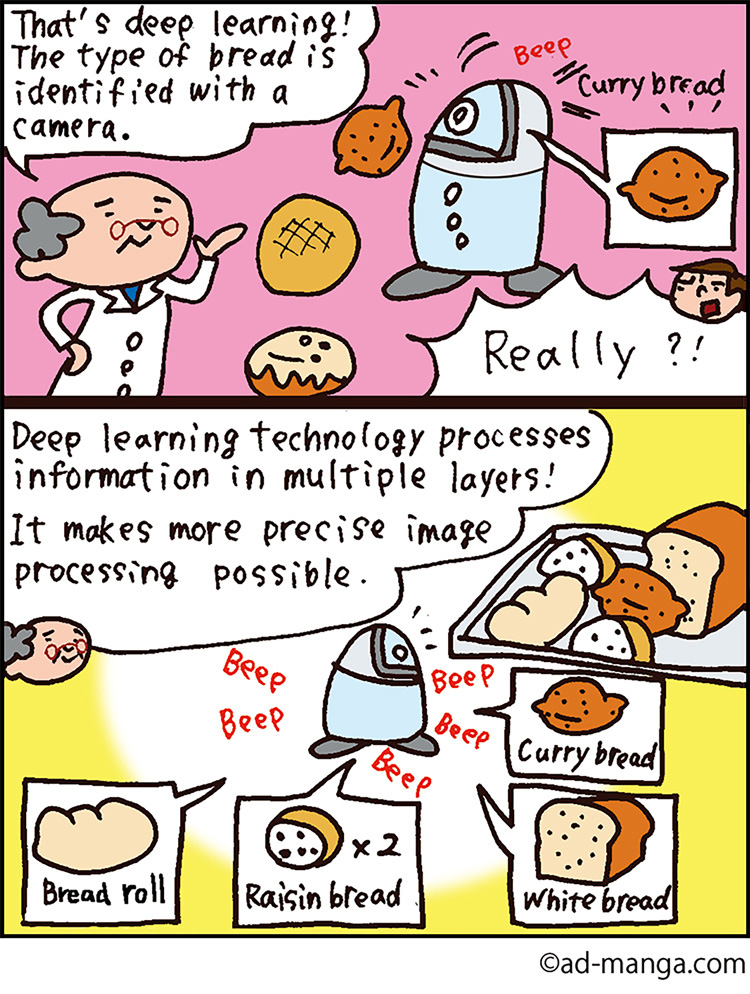
Just as babies learn words, AI itself learns and makes judgments
Do you think that AI has become increasingly smart in recent years? AI helps make our lives convenient with a variety of services such as the voice assistants and automatic translation available on smartphones.
It is said that advances in AI such as these have been brought about by deep learning, a term we have often heard in recent years. It is a type of machine learning that uses a neural network. Deep learning is a method aimed at deriving an optimal solution from a machine that is repeatedly trained using a large amount of data. A neural network is a machine-learning method intended to mimic the mechanism by which neurons in the brain transmit information. Given an enormous amount of data consisting of questions and correct answers, a neural network will learn relationships between the questions and correct answers and will be able to give the correct answer to a new question.
This is like babies who learn words by hearing their parents speaking them repeatedly. For example, parents repeatedly say to their babies, “Look, it’s a doggy” until the baby can identify a dog. At first babies say “doggy” when they see a cat, but after seeing many dogs and cats they learn to differentiate them.
The word “dog” includes animals that are quite different in color, size, body shape, hair length, etc., and yet we can tell whether the animal before us is a dog. This is because we have seen many dogs and have a knowledge of their characteristics. So the mechanism of deep learning is very similar to how babies learn the names of things.
Extracting relationships from large amounts of labeled data using multilayer neural networks
Having the largest possible pool of questions and answers is the key to deep learning. It would be meaningless, though, to have plenty of questions without answers, or to have plenty of images without tags. In other words, using the example above, learning is possible only when there are a large number of dog images with labels.
With conventional AI, taking a tomato as an example, it was necessary to tell the computer each characteristic, such as the color, roundness, and green stem. However, teaching all the characteristics is not a feasible method because some tomatoes are yellow and others are not round.
In that respect, deep learning has the revolutionary capability of extracting features by itself. Drawing features from a large amount of image or voice data, which can now be easily acquired (but previously was difficult to get), deep learning can answer questions.
Using a multilayered neural network, it can learn from a given set of data, extract features, and recognize complicated information.
The input image data is analyzed down to the individual pixel level and classified into “layers” for color, shape, and more complicated features. The data in each layer is connected to the data in the next layer. From these many layers, complicated features are extracted based on combinations of color, shape, etc. The term “deep” refers to the depth of these layers.
The main reason that it is possible to analyze data in multiple layers in this way is the dramatic increase in the computational ability of computers. Today’s personal computers have a processing capability equal to that of supercomputers in the past. The accuracy of feature extraction, which requires a large amount of data processing, has also improved rapidly.
More applications for consumers as vast amounts of data become readily available
Typical applications of deep learning are smart speakers with voice recognition and self-driving cars that recognize the surroundings based on information from cameras and GPS. In addition, deep learning is used in various fields such as web search technology and automatic photo tagging on social networking sites. These functions and services, which are available because large amounts of labeled data can be acquired easily via the Internet, are mainly for consumers.
Some efforts to use deep learning for industry are underway, but the applications and necessary data vary according to the industry or company. Unlike the situation for consumers, it is difficult to collect a large enough amount of labeled data to operate a service because the volume of available data is limited. In order to solve this problem, research on algorithms that can successfully extract features from a small amount of data is underway.
As computer processing capability further improves in the future, it may become possible to solve more complicated problems. Or, perhaps a type of AI using a totally different mechanism will appear. As the use of AI in creasingly becomes a part of our lives, we have to wonder what future developments in AI will bring.